The Hard Truth About AI in Supply Chains: Barriers to Effective Integration
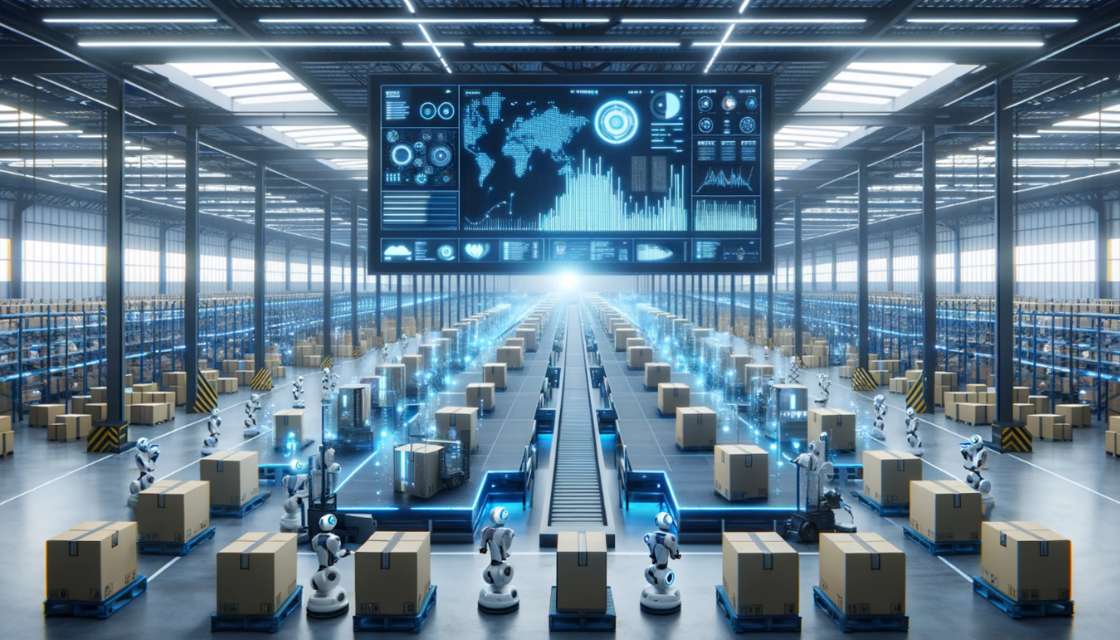
The emergence of generative AI applications, such as ChatGPT, has brought artificial intelligence (AI) into the spotlight. While this surge in interest is notable, AI-based digital tools have long aided supply chain planners in enhancing decision-making across various operations, including process automation, route optimization, warehouse management, and quality control.
Despite the evident benefits, the pace of digital tool adoption within the supply chain sector has been gradual. A significant finding from a recent survey on digital adoption in supply chain planning reveals that only about 40% of planners have transitioned to using new technology tools, even though adopting them generally improves planning outcomes. Interestingly, 93% of planners expressed a willingness and readiness to adopt new technologies.
Organizational Change Management in AI Adoption
As supply chain operations integrate more AI-driven tools, the transition can disrupt established processes and roles, creating a need for change management to navigate this transformation smoothly and efficiently.
A major hurdle in this transition is the disruptive process of dismantling long-optimized legacy systems to integrate AI-driven processes. Challenges often arise in syncing new software with existing ERP systems, requiring customized solutions to handle real-time data across various supply chain functions. This disruption can affect daily operations and necessitates a steep learning curve for operational teams.
Furthermore, automation demands significant changes in workforce skills. Beyond basic training, employees need upskilling in data literacy and AI applications. For example, procurement managers accustomed to traditional methods may need to learn how to use AI-driven predictive analytics for forecasting supply issues or price fluctuations. This upskilling may require targeted educational programs and the recruitment of new talent with advanced technical skills, which adds complexity to workforce management.
Additionally, the recent hype around generative AI has fueled fears of job replacement by AI, despite little evidence supporting this outcome. These fears often lead to resistance against adopting AI technologies, driven by concerns about job security and the potential for AI to render existing skills or roles obsolete. Overcoming this cultural hurdle necessitates a tactful approach from management. This includes consistent reassurance, engagement, and transparency to help employees view technological advancements as allies rather than adversaries.
Technical Hurdles in AI Integration
Disparate, inconsistent data sets scattered across various platforms challenge many organizations, complicating AI integration. For instance, a supply chain manager might struggle to forecast demand effectively when dealing with inventory, sales, and customer feedback systems that all use different data standards. This situation necessitates sophisticated data harmonization techniques, substantial IT resources, and potential delays in AI deployment.
Issues of system compatibility and scalability further complicate integration. Legacy systems, crucial to many current operations, typically lack the flexibility required by rapidly evolving AI technologies. For example, an automotive manufacturer’s legacy system, which updates at the end of each work shift, will struggle to integrate with AI-driven predictive maintenance tools that require real-time data. This often necessitates costly upgrades or the development of custom solutions to bridge the gap between the old technology and new, data-intensive AI applications.
Additionally, the lack of data format and protocol standardization poses significant barriers. For example, a global retail chain may have different inventory system standards in North America versus Europe, complicating the implementation of a global inventory management AI. Ensuring seamless integration across the supply chain requires extensive stakeholder coordination and standardized protocols.
Economic and Security Challenges in AI Integration
While addressing integration and standardization challenges is crucial, organizations must also consider the significant financial and operational implications associated with implementing AI technologies in supply chain management.
The initial cost for AI technologies is often high, encompassing not just the purchase of software and hardware but also the construction of necessary infrastructure. Such infrastructure includes robust servers for processing large datasets, advanced analytics tools, and scalable cloud storage solutions, representing a significant capital investment that can be prohibitive for smaller organizations.
Operational costs also play a critical role. Running AI systems involves ongoing expenses like maintenance, updates, and technical support, alongside continuous training for personnel. These costs can accumulate, impacting the overall return on investment and potentially slowing the adoption rate of AI technologies within the supply chain.
Moreover, as supply chains incorporate more AI and data analytics, concerns about data privacy and security become more prominent. Ensuring the security of sensitive information, including proprietary business data and customer details, is crucial, as breaches can lead to severe financial and reputational damage. The challenge extends to maintaining compliance with evolving data protection regulations, which can vary significantly by region and require constant vigilance and adaptation.
—————————
Since 1995, AmTech has been innovating supply chain solutions with high-quality gears, shafts, castings, and more. Our advanced manufacturing processes ensure efficiency and resilience across various industries. Discover more about AmTech’s products and services.
References: